Oil and Gas 4.0
Refineries are one of the sectors that have adopted the term “Industry 4.0”, giving place to “Oil and Gas 4.0”, which main goal is the implementation of digitalisation, automation, Machine Learning (ML) and Big Data technologies to enhance the capabilities of the sector and consequently, reduce costs and maximise profit.
Nowadays, we are immersed in a new industrial revolution, named as “The fourth Industrial Revolution” or “Industry 4.0” in which the introduction of new breakthrough technologies has achieved unprecedent rates of productivity and efficiency. Among the sectors that have adopted the Industry 4.0 philosophy, refineries are one of them, giving place to “Oil and Gas 4.0” which core goal is to achieve higher value by employing digital technologies. The Oil and Gas industry is complex and diverse, and it can be divided into three different sectors depicted in Figure 1: upstream, midstream and downstream.
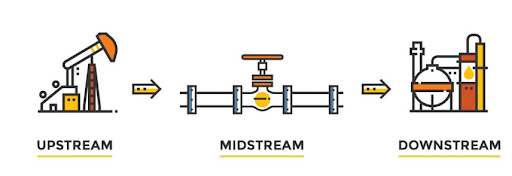
“Upstream” refers the exploration and production of oil and natural gas. The “midstream” segment is related to the transportation and storage of crude oil and natural gas. The final sector, known as “downstream”, involves the conversion of the crude oil and natural gas into thousands of marketable subproducts. Such conversion is done in the refinery, which is a group of manufacturing plants composed of several unit operations that convert raw crude into valuable subproducts.
Refineries already produce vast volumes of subproduct. However, the direction of the refining industry is determined by 1) increased operating costs or investments due to stringent environmental regulations and 2) the accelerating globalisation resulting in stronger international petroleum price scenarios [1]. Therefore, the optimization of the refinery operations to reduce costs and improve efficiency is mandatory to achieve the difference between profit and loss and be globally competitive. In this regard, the digitalisation in the refinery sector is essential to cope with future challenges and achieve a profitable outcome [1].
In fact, the digitalization in the Oil and Gas sector has become a reality by the development of faster and more informative sensing technology able to collect and store information from multiple sources, as well as the increment of computational resources capable of process large amount of data by advanced analytical tools. In this context, refineries are already collecting data from process units and streams. This data is related to flow, temperature, pressure, conductivity, PH, and so on. Therefore, they have collected massive amounts of data yielding to data-rich scenarios. However, most of the refineries are in a “data-rich and information poor” scene, i.e they are rich in data but need processes to extract meaningful information and create advantage from this data. For doing so, data analytics (Artificial Intelligence (AI) and ML) are relevant technologies to learn and extract hidden patterns from the data.
In this regard, most of the important AI applications in the refining plants involve the development of “soft-sensors”, which can infer any system variable based on some other related variables [2,3]. They complement hardware sensors, suppose a low-cost alternative and estimate data in real time. Among the AI applications in the Oil and Gas industry, there are some relevant ones regarding the optimal scheduling for crude oil loading and unloading [4], or steal injection [5], multi-level production planning [6], fault [7] or leakage detection system [8] and maintenance decision of petrochemical plant [9]. In addition, the refinery sector must comply with different standards and strict regulations. Consequently, AI applications for subquality product estimation are of outmost importance in this sector.
Traditionally, the way to measure the subproduct quality is by online analysers or laboratory tests. The online analysers consist of physical sensors with an automatic sample acquisition tool for performing the quality analysis. However, they are usually performed once the refining process ends, which can delay the possible corrective actions. Similarly, the laboratory tests have also a delay between the sample collection and the results, generally of hours. In order to circumvent it, soft-sensors are designed and developed to complement current instruments, enhance the flexibility and resilience of industrial systems and improve the productivity of the industry. Until the date, several soft-sensors that have been developed to supplement diverse laboratory tests, such as 95% ASTM-D86 of naphtha, gas oil and kerosene [10–13], 10%, 50% and 90% boiling points of diesel [14] and the carbon decomposition rate [15], among others. Online analysers are also complemented by recently developed soft-sensors designed to estimate the octane number in gasoline in a power-former unit [16], the side-cut and overhead stream in a desihexanizer column [17], toluene estimation [18] or the butane content on the bottom of a debutanizer column [19]. Nevertheless, there are still many challenges in which soft-sensors can be developed to support refinery plant operators by providing instant access to important information. Some of these challenges consists of improving the energy use and detecting efficiency losses in the plants.
Author:
Diana Manjarrés
Tecnalia, Basque Research & Technology Alliance (BRTA)
http://www.tecnalia.com
References
[1] James G Speight. The refinery of the future. Gulf Professional Publishing, 2020.
[2] Luigi Fortuna et al. Soft sensors for monitoring and control of industrial processes. Vol. 22. Springer, 2007.
[3] Ali Al-Jlibawi et al. “The efficiency of soft sensors modelling in advanced control systems in oilrefinery through the application of hybrid intelligent data mining techniques”. In: Journal of Physics: Conference Series. Vol. 1529. 5. IOP Publishing. 2020, p. 052049.
[4] Georgios KD Saharidis, Michel Minoux, and Yves Dallery. “Scheduling of loading and unloading of crude oil in a refinery using event-based discrete time formulation”. In: Computers & Chemical Engineering 33.8 (2009), pp. 1413–1426.
[5] Leonid Sheremetov, Jorge Martınez-Muñoz, and Manuel Chi-Chim. “Two-stage genetic algorithm for parallel machines scheduling problem: Cyclic steam stimulation of high viscosity oil reservoirs. In: Applied Soft Computing 64 (2018), pp. 317–330.
[6] Sandeep Singh Chauhan and Prakash Kotecha. “An efficient multi-unit production planning strategy based on continuous variables”. In: Applied Soft Computing 68 (2018), pp. 458–477.
[7] P Gil et al. “Data Anomaly Detection in Wireless Sensor Networks with Application to an Oil Refinery”. In: 2018 13th APCA International Conference on Automatic Control and Soft Computing (CONTROLO). IEEE. 2018, pp. 425–429.
[8] Peng Xu, Rui Du, and Zhongbao Zhang. “Predicting pipeline leakage in petrochemical system through GAN and LSTM”. In: Knowledge-Based Systems 175 (2019), pp. 50–61.
[9] Bin Zhao et al. “Maintenance decision methodology of petrochemical plant based on fuzzy curvelet neural network”. In: Applied Soft Computing 69 (2018), pp. 203–212.
[10] Luigi Fortuna, Salvatore Graziani, and Maria Gabriella Xibilia. “Comparison of soft-sensor design methods for industrial plants using small data Sets”. In: IEEE Transactions on instrumentation and measurement 58.8 (2009), pp. 2444–2451.
[11] Lukasz Pater. “Application of artificial neural networks and genetic algorithms for crude fractional distillation process modeling”. In: arXiv preprint arXiv:1605.00097 (2016).
[12] Bahareh Bidar et al. “A data-driven soft-sensor for monitoring ASTM-D86 of CDU side products using local instrumental variable (LIV) technique”. In: Journal of the Taiwan Institute of Chemical Engineers 84 (2018), pp. 49–59.
[13] Yajun Fan et al. “A Data-Driven Soft Sensor Based on Multilayer Perceptron Neural Network with a Double LASSO Approach”. In: IEEE Transactions on Instrumentation and Measurement (2019).
[14] Yalin Wang, Dongzhe Wu, and Xiaofeng Yuan. “A two-layer ensemble learning framework for data-driven soft sensor of the diesel attributes in an industrial hydrocracking process”. In: Journal of Chemometrics 33.12(2019), e3185.
[15] Wang Yuqiao et al. “A soft sensor for carbon content of spent catalyst in a continuous eforming plant using LSSVM-GA”. In: Proceedings of the 31st Chinese Control Conference. IEEE. 2012, pp. 7056–7060.
[16] Luigi Fortuna et al. “Virtual instruments based on stacked neuralnetworks to improve product quality monitoring in a refinery”. In: IEEE Transactions on Instrumentation and Measurement 56.1 (2007), pp. 95-101.
[17] Srečko Herceg, Željka Ujević Andrijić, and Nenad Bolf. “Development of soft sensors for isomerization process based on support vector machine regression and dynamic polynomial models”. In: Chemical Engineering Research and Design 149 (2019), pp. 95–103.
[18] Ivan Mohler, Željka Ujević Andrijić, and Nenad Bolf. “Soft sensors model optimization and application for the refinery real-time prediction of toluene content”. In: Chemical Engineering Communications 205.3 (2018), pp. 411–421.
[19] Xiaofeng Yuan et al. “Deep learning-based feature representation and its application for soft sensor modeling with variable-wise weighted SAE”. In: IEEE Transactions on Industrial Informatics 14.7 (2018), pp. 3235–3243.